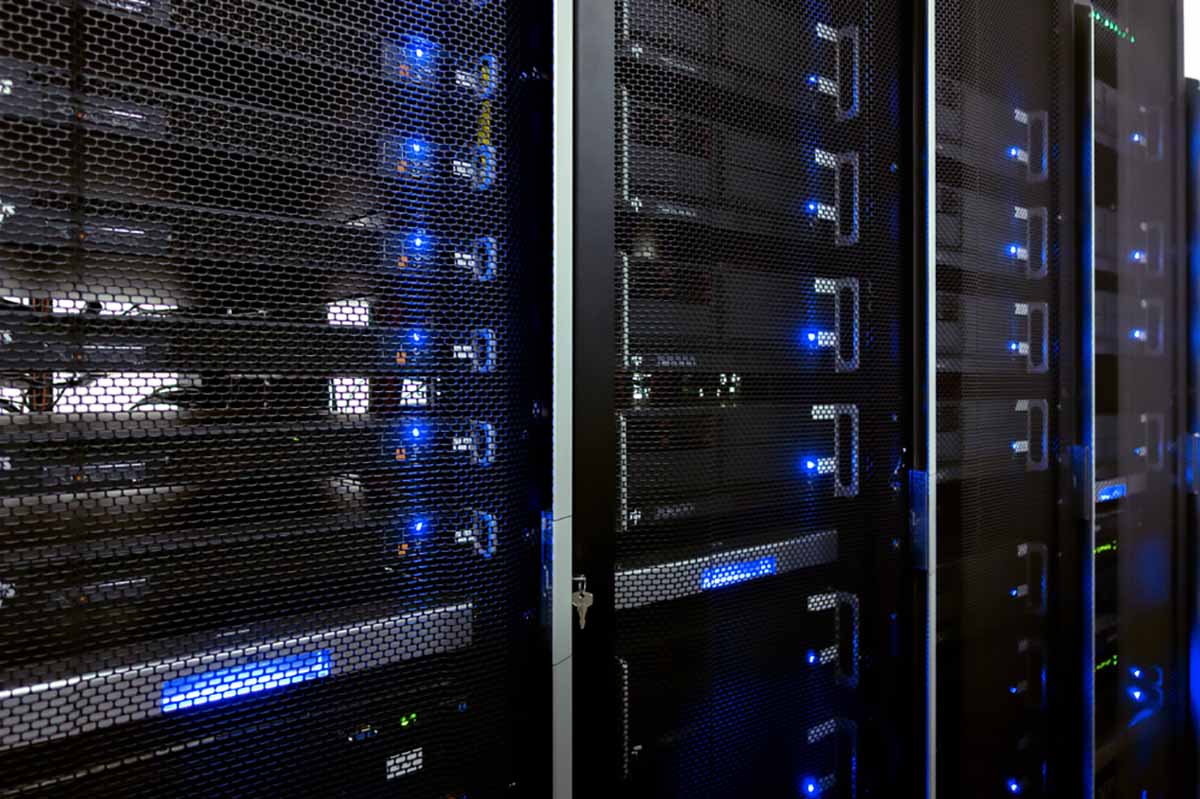
A new paper in Nature Computational Science examines potential scenarios for AI-related e-scrap generation, which is driven by its data center needs. | Funtap/Shutterstock
As artificial intelligence continues to ramp up, researchers said the computing-heavy tool could lead to skyrocketing volumes of end-of-life electronics and called for equal attention to asset management.
Researchers from the University of China Academy of Sciences in Beijing, Reichman University in Israel and the University of Cambridge in the U.K. on Oct. 28 published “E-waste challenges of generative artificial intelligence,” which appeared in the peer-reviewed Nature Computational Science journal.
The research traces the growth in large language models, the type of AI that’s used in high-profile tools like ChatGPT. These tools are “trained on vast datasets,” the researchers noted, demanding “considerable computational resources for training and inference, which require extensive computing hardware and infrastructure.”
In practice, that means more – and much more powerful – data centers. The researchers considered waste generation from 2023 through 2030 under a few different models for how aggressively AI could be rolled out. They looked only at the key hardware involved in AI computing: servers that include graphics processing units, central processing units, storage, memory units, internet communication modules and power systems.
Without any strategic planning, the research found cumulative e-scrap generation of these materials from data centers could total at least 1.2 million metric tons under the most limited rollout and up to 5 million metric tons under the most aggressive.
This e-scrap generation would naturally be concentrated in data center-heavy regions, with 58% in North America, 25% in East Asia and 14% in Europe, the researchers added.
In a statement to E-Scrap News, study author Penn Wang of the Chinese Academy of Sciences said the findings underscore a need for greater transparency from data center operators on how much end-of-life material they are generating, a need to better link data center operators with electronics processors, greater regulation on how end-of-life material from data centers is handled, and global cooperation to handle these projected volumes of material.
AI’s energy consumption draws wide focus
As AI has exploded into the public discourse over the last couple years, generating both excitement and trepidation for its potential, many analysts have considered the ripple effects of major data center growth.
Early this year, Goldman Sachs projected AI will increase data center power demand by 160% by 2030, because “a ChatGPT query needs nearly 10 times as much electricity to process as a Google search.”
That power consumption means more emissions, the World Economic Forum said in July, noting that data center demand had contributed to a 30% spike in Microsoft’s CO2 emissions and a 50% spike in Google’s. The energy consumption challenge has led to ambitious planning, including Microsoft recently proposing to revive the infamous Three Mile Island nuclear power generator in Pennsylvania.
Now the research into waste generation is highlighting the need for similarly ambitious planning to reduce the projected growing volume of end-of-life devices from AI infrastructure.
“This rapid growth in hardware installations, driven by swift advancements in chip technology, may result in a substantial increase in e-waste and the consequent environmental and health impacts during its final treatment,” the researchers wrote.
IT asset disposition firms are already gearing up to meet this projected influx. But the new research offers insights into how important data center refresh practices will be during the next several years.
Extending first-use and prioritizing reuse are key
The Nature Computational Science study looked at several scenarios that could affect the volume of end-of-life data center assets, and they found the most effective practices are extending equipment’s first use and prioritizing reuse through dismantling and parts harvesting.
First-use extension can be achieved when a data center operator moves an end-of-life piece of equipment into a lower intensity application. The paper offered the example of a server at the end of its three-year lifespan being redeployed into a section of the data center serving non-AI or less-intensive AI needs.
Redeploying assets in this way for just one additional year would reduce equipment entering the end-of-life stream by 62%, or 3.1 million metric tons, under the “aggressive” AI adoption scenario.
Engaging in server module reuse by dismantling, refurbishing and remanufacturing used GPU or CPU modules and returning them back into the high-intensity AI applications, for example, would reduce waste generation by 42%, or 2.1 million metric tons, the researchers estimated.
There are plenty of other factors that will influence end-of-life server generation. The researchers noted geopolitical factors like chip import and export restrictions prevent data centers in certain countries from obtaining the latest-model equipment. That’s important because newer models can often perform better with less physical equipment.