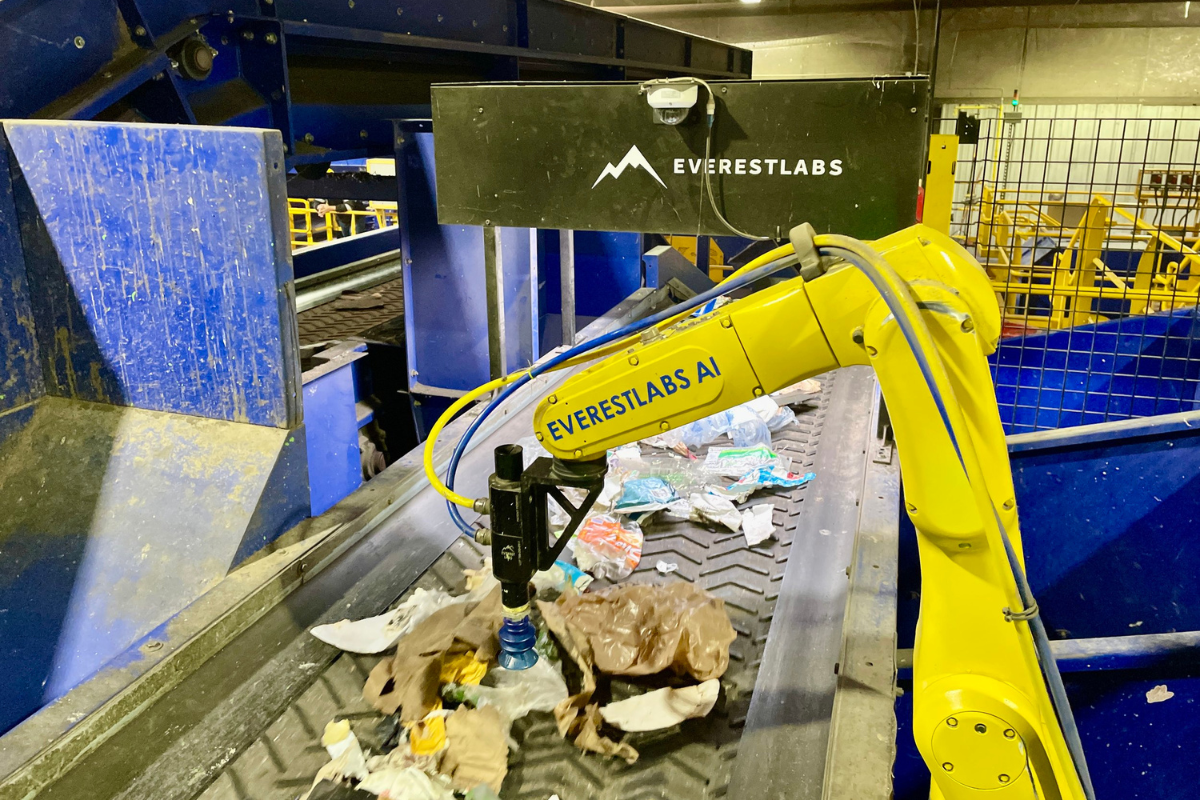
The data gleaned from AI imaging systems can help improve MRF operations on a wider scale. | Photo courtesy EverestLabs
Equipment supplier EverestLabs recently highlighted a handful of examples of how artificial intelligence-equipped data analytics can help MRF operators modify equipment settings to improve commodity recovery.
Fremont, California-headquartered EverestLabs is one of several companies outfitting recycling facilities with robotic arm sortation equipment, machine learning imaging systems and a software platform to make sense of all the captured data.
In a March 27 presentation, the company described how its equipment is helping MRFs and a plastics reclaimer improve material recovery, sometimes in unexpected ways because of the new data available to the facility operators.
Data insights facilitate upstream equipment tweaks
Up in Chicago, a large MRF operated by Lakeshore Recycling Systems is using AI and robotics to specifically target increased UBC recovery. The facility, which is called The Exchange and opened in 2023, processes about 500 tons per day.
The MRF operator last year installed EverestLabs equipment funded by a revenue share program with the Can Manufacturers Institute. The funds went towards a robotic sorting arm on the last-chance line, across from the UBC bunker, and the robot began grabbing cans from the residue line.
The company also installed the AI image system, called RecycleOS, and the analytics from that equipment provided some particularly valuable insights.
“They were able to deduce that the issues with all the UBC ending up on the last chance line was actually due to their eddy current underperforming,” said Katherine Nellis, solutions engineer at EverestLabs. “This data allowed them to justify the necessary equipment needs to fix and to tune that eddy current so that it became more effective at actually diverting the UBC out of the stream.”
The end result was far fewer cans even making it to the last-chance line for the robotic arm to pick. With fewer picks per minute of UBCs, the equipment supplier was able to dial back the robot’s UBC focus and have it target a wider spread of materials, including natural HDPE containers.
One year on, Nellis reported the robot recovered 1.3 million UBCs in 2024 and is recovering about 600,000 cans per quarter.
“It shows how powerful the data can be in allowing MRF operators to improve not just what is happening on the sort line, where the camera is, but make improvements all throughout the MRF, using that data to actually calibrate and tune their other equipment,” Nellis said.
Similarly, a Pacific Northwest MRF implemented EverestLabs’ AI vision system on its last-chance line, and after a month of data collection, it showed the facility was losing $43,300 per month in commodity value.
The plant manager used that data to make changes upstream in the sorting facility, including adjusting settings on an eddy current separator and optical sorter and adding a manual sorter on the aluminum line.
These changes helped the facility capture an additional $28,300 per month, or $336,000 per year.
“This data helps them continually optimize their machinery, enabling them to be more profitable and divert what would go to landfill back into the circular economy,” said Makenna Phillips, marketing specialist at EverestLabs.
Quantifying dollar value of a particular conveyor line
Across the country, a MRF in the mid-Atlantic region processing 170 tons per day found the equipment helpful in lowering operating costs.
“It’s no shocker to anybody in the industry that labor is one of the highest costs in a MRF, and this particular MRF had been struggling with labor sourcing and high turnover since the pandemic,” Nellis said.
The facility was set up to have a flat conveyor at the end of its material flow, acting partly as a container line and partly as a last-chance line. Historically, the MRF staffed the line with five manual sorters, Nellis said.
After installing the AI image system at the front of the line, the MRF got a full picture of which materials were making it to the last-chance/container line. And with the knowledge of how many UBCs, PET and HDPE containers and other specific commodities were hitting the line, the facility was able to calculate the materials’ value.
“What they found was that there actually wasn’t enough material value there to justify the five sorters to stand there and actually recover it,” Nellis said.
The facility opted to install robotics on that last-chance line instead, finding that the equipment was able to maintain similar material capture with lower ongoing costs.
Monitoring PET contamination in real time
Outside the MRF realm, a PET reclaimer had already been using EverestLabs robotics to pull out contaminants on the clear and green PET quality control lines. But the company wanted to find new ways to improve other areas of plant operations.
“In a plastics recycling plant, they’re all about having a high throughput, which is how much material they’re processing, but at the same time, it’s important that they maintain a high yield on the output of the PET flakes and recycled content that then they are sending out to their customers,” Nellis said.
The company began generating a metric of “contaminants per minute” on the quality control lines, and it was able to send those numbers in real time to an analytics tool the facility uses with other machinery, including wash lines, Nellis said.
“Those machines are greatly impacted by the purity of the stream that enters it,” she said. The reclaimer has specific contamination thresholds that have to be met for different equipment, and it’s now able to use the contaminants-per-minute analytic to ensure those thresholds are met.
“They can stop the line, or they can divert the stream from going into the wash process and perform additional quality control on it to make sure that it’s optimal for further recycling,” Nellis said. “This really just allows them to have better control over the quality of their end feedstock.”