This article originally appeared in the Fall 2020 issue of E-Scrap News. Subscribe today for access to all print content.
Recently, a new wave of robotic automation has generated incredible ROIs for many industries.These technological trends – with some adaptations – could soon be opening the door to new operational possibilities and profits in the e-scrap and ITAD sectors.
This article will explain the general details around the evolution in robotics and “machine learning,” showing the specific applications the technology can have for companies that recover, refurbish and remarket electronics.
Flow of the future?
Imagine the following scenario happening inside an ITAD or e-scrap facility.
A laptop that enters the site is isolated and placed in a box, and cameras installed inside take photos of the asset. The box’s processing unit examines the digital images to evaluate and determine the laptop’s model, type, configuration, cosmetic grade, and any other important information. All of this data is automatically entered into the company’s ERP system. At this point, a decision-making algorithm, using the latest pricing information, decides which route the laptop should take downstream for maximum ROI.
An autonomous mobile robot, or AMR, then moves the device to the facility’s testing/wiping room and begins that process. Finally, the AMR moves the laptop into the designated area for refurbishing, parts harvesting or recycling.
Meanwhile, on the sales side, an ad for the device – and all others moving through the facility – is automatically generated using templates and sent to relevant websites or email sales lists. When the item is sold, an AMR retrieves the device and delivers it to a packaging machine.
The laptop has been assessed, processed and shipped, all without a human lifting a finger.
Of course, there is a wide variety of possibilities for robotics within electronics recovery operations, and the best solution for each operation will be different. But what the above scenario illustrates is that robotic developments can add remarkable efficiencies to a processing site.
AI, machine learning and deep learning
So what are the specific innovations in technology that are allowing us to even imagine a reality like the one outlined above?
To understand, we need to know the distinctions between three terms: artificial intelligence, machine learning and deep learning.
Artificial intelligence (AI) is a broad area of computer science with the goal of creating machines that can behave intelligently. Machine learning is a subfield of AI in which machines develop a model of how to behave by learning from examples. Finally, deep learning is a modeling technique used in machine learning. In deep learning, models represent what is being modeled through multiple layers of abstraction.
Innovations in deep learning have been the engine of progress at the heart of many technological advances, including autonomous vehicles, Amazon’s Alexa and the growing array of robotic sortation devices now being deployed in municipal recycling facilities that handle paper, plastics and other common recyclables.
Machine learning and deep learning are revolutionary because using these techniques, all a programmer needs to do to help a machine learn to do a task is to show it a lot of examples of correct behavior. How, for example, do you come up with rules to grade the cosmetics of a device or tell the robot where to grab a device when handling it? With machine learning, you can do this by showing the software what grade similar devices have received or giving examples of proper handling.
Of course, when you show examples, you cannot show all possibilities that exist and, indeed, if an example is very different from those used to train the machine, then it may stumble. This is not all that different from the process of training a human. With both a human or a machine in this scenario, the operator simply needs to show the specific steps needed to handle this novel example. After enough examples have been presented, one can be confident that the machine will adapt for these novel scenarios as well.
Adaptation by robots is indeed amazing, but the process of adaptation in an ongoing operation could turn out to be very expensive. For example, if we are using a pick-and-place robot that is powered by machine learning, it may see a device it has never encountered before, pick it up clumsily, and damage the device.
This is why it’s very important to know the scope that the machine can work in and make sure its error rate is acceptable. In addition, you need to make sure you have a process in place to handle the task outside of the scope. This is the type of work that will guide the development of AI in electronics recycling and reuse in the years ahead.
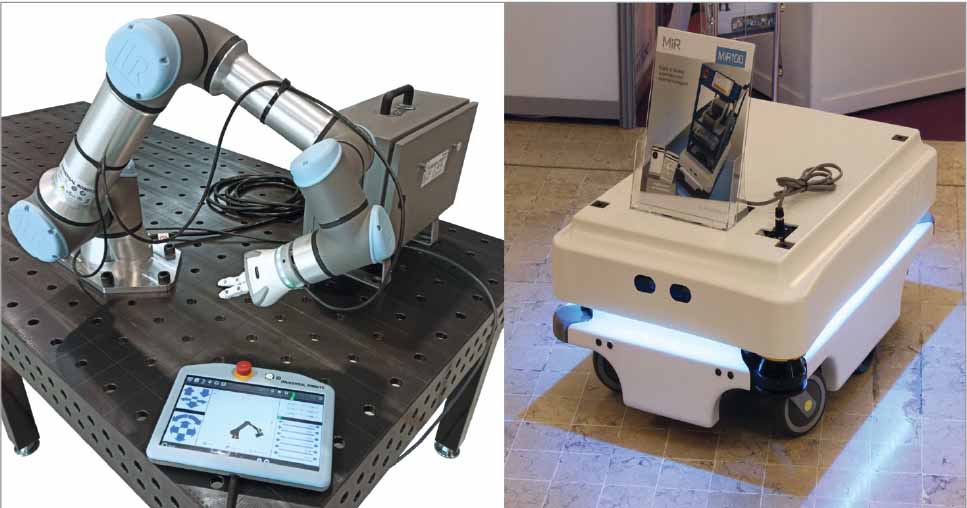
A cobot (collaborative robot), shown on the left, can work alongside humans with no cage. An autonomous mobile robot, shown on the right, can roam safely inside a warehouse with no special hardware installed.
Drilling down deeper
Now that we have an understanding of the broad concepts governing the rapid advancement of robotics today, let’s get a bit more granular. There are a number of important trends within the machine learning space itself that are opening up new possibilities within robotics.
It will be useful to know exactly how these more specific tech developments can be applied to the e-scrap and ITAD sectors.
First is machine vision, a term used to describe technologies that let machines understand images and videos. Machine vision has been one of the biggest beneficiaries of deep learning, and in the electronics processing arena, it holds great potential for automating inventory and cosmetic grading practices.
Through machine vision, it is possible to create an automated system that can identify the brand, model, cosmetic condition and virtually any other characteristic of an asset through examination of a digital image of the device. The immediate benefit of this is that your inventory speed goes up while the error rate is reduced.
There are many other potential benefits to such a system, including standardizing grading practices across the industry. Automated grading systems used by different processors would produce images allowing a third party to verify the conditions of a device or settle disagreements. As long as the buyer trusts the third party, they will also trust that you own the advertised device. This opens up the door to many potential buyers who may not have come forward if they didn’t have this sense of clear, documented verification.
Furthermore, if such a system was adopted industry-wide, online marketplaces would become the preferred wholesale transaction channels, making it possible to establish industry-wide pricing databases. These databases could help each operator decide – based on data – what to do with each device for a maximum ROI.
Another important trend to discuss is the agility and variation in robotics. For electronics processors, this carries repercussions in realms such as material movement, wiring, packaging and shredding.
The field of robotics is being reshaped with respect to hardware, software and how one can buy these tools. On the software side, deep learning is making robots more flexible than ever, so you can expect them to be able to pick, place and manipulate a wide variety of devices. On the hardware side, a new generation of robotic arms called “cobots” or collaborative robots, are designed for direct human/robot interaction within a shared space.
What this means is that the machines stop upon contact. They are not caged and are easy to program, even for a non-programmer. All of these features make them suitable for the complex, ever-changing workflow of electronics recovery. Cobots can be used to attach barcodes, shred hard drives, and package and place devices on pallets. Since they are not caged, they can be easily moved around the warehouse multiple times a day and put to use for different applications.
This also relates to the autonomous mobile robots (AMRs) touched on earlier. AMRs are the new generation of automatic guided vehicles (AGVs). AMRs can be used without any of the supporting infrastructures that AGVs needed, such as markers, wires, magnets implanted in the floor, or precisely located laser targets. These devices are designed to avoid obstacles and will stop when they see a human in their path. So, instead of putting conveyor belts in your warehouse and losing flexibility to your changing demands, AMRs will allow you to divide a warehouse into “islands of work,” with the robots moving goods among them as needed.
Talk it up
Another notable advancement arising from deep learning is the increase in a machine’s ability to comprehend and generate text. Despite this progress, a computer’s grasp of language is still far below that of a child.
But there has been more success with applying natural language processing (NLP) as a tool for text summarization rather than text generation. This has the possibility of enhancing an e-scrap operator’s abilities in developing sales templates and bolstering communication with clients and partners.
For example, this technology could help an operator by automatically summarizing inbound inventory list emails, allowing the operator to quickly see what material is available across the board and look for better opportunities.
NLP can also be used to generate sales templates. Considering the current state of the art, however, you can expect to see higher ROI with just using common sales templates and automated scripts. That being said, NLP is a rapidly advancing technology, and soon, things may be very different as seen with gigantic pre-trained models such as GPT-3 of OpenAI.
Another common use of NLP is in chatbots. The goal of a chatbot is to automate conversations. Given the current realities of the technology, using a chatbot as a sales tool has not usually been very successful. A more rewarding approach has been to use them as a search tool within large FAQ databases or augmenting customer service with automated retrieval of relevant information for faster and more accurate responses.
A final trend to keep in mind across the field of artificial intelligence is robots-as-a-service – basically, the notion of a subscription model, which has gained popularity in the field over the past few years.
The vendor will provide you with a service and you only pay for what you use. If provided equipment breaks down, the vendor is responsible to keep the designated part of your operation up and running. Since there is almost no upfront cost, the model removes the automation barrier for small- and mid-size operations. Furthermore, since you are not purchasing equipment, all costs come from operating expenses. This means that you will almost immediately realize a return on your investment and enjoy a faster purchasing cycle.
A gradual evolution
The new generation of automation can increase an operator’s efficiency in sales, material handling and inventory processes. It also has the potential to increase industry-wide standardization and trust. These possibilities are mostly fueled by machine learning, and machine learning-powered products are a great choice when we are faced with open-ended circumstances, which is the reality of the ever-changing material stream in electronics processing.
While the concept of automation is very exciting, one needs to be aware of the risk of incorporating too much automation too quickly. Doing so can introduce “brittleness” to the operation. A better approach would be to automate gradually, starting with the “low-hanging fruit” before moving on to more complicated tasks. Consulting with an automation expert can definitely help to identify the best opportunities.
And like so much else, automation within the sector can be pushed ahead through data sharing and open dialogue among stakeholders. At Numadex, we are currently running a survey on the state of automation in the electronics processing industry. Add your voice at numadex.com/survey or using the QR code above. Once the responses have been tabulated, we can let you know where you stand among your peers.
Iman Jenabzadeh is the founder and CEO at Numadex, which specializes in remote-controlled and AI robotics services. He can be contacted at [email protected], and readers can sign up for the company’s newsletter at numadex.com.
The author would like to thank the following industry members for their feedback during the development of this article: Robert Houghton, CEO of Sage Sustainable Electronics; Patty (Osterberg) McKenzie, director of outreach at SERI/R2; Elnaz Nouri, senior scientist at Microsoft; and Amir Nader Ghazvini, data scientist at Abzooba.